In this blog article, you will learn the difference between AI vs ML vs DL. or you can say the difference between Artificial Intelligence vs Machine Learning vs Deep Learning.
Artificial Intelligence
Artificial Intelligence is basically the mechanism to incorporate human intelligence into machines through a set of rules(algorithm). AI is a combination of two words: “Artificial” meaning something made by humans or non-natural things and “Intelligence” meaning the ability to understand or think accordingly. Another definition could be that “AI is basically the study of training your machine(computers) to mimic a human brain and its thinking capabilities”.
AI focuses on 3 major aspects(skills): learning, reasoning, and self-correction to obtain the maximum efficiency possible.
Based on capabilities, AI can be classified into three types:
- Weak AI
- General AI
- Strong AI
You can check our article on the “Difference between Artificial Intelligence and Machine Learning.”
Machine Learning
Machine Learning is basically the study/process which provides the system(computer) to learn automatically on its own through experiences it had and improve accordingly without being explicitly programmed. ML is an application or subset of AI. ML focuses on the development of programs so that it can access data to use it for themselves. The entire process makes observations on data to identify the possible patterns being formed and make better future decisions as per the examples provided to them. The major aim of ML is to allow the systems to learn by themselves through the experience without any kind of human intervention or assistance.
It can be divided into three types:
- Supervised learning
- Reinforcement learning
- Unsupervised learning
You can check our article on the “Difference between Artificial Intelligence and Machine Learning.”
Deep Learning
Deep Learning is basically a sub-part of the broader family of Machine Learning which makes use of Neural Networks (similar to the neurons working in our brain) to mimic human brain-like behaviour. DL algorithms focus on information processing patterns mechanism to possibly identify the patterns just like our human brain does and classifies the information accordingly. DL works on larger sets of data when compared to ML and the prediction mechanism is self-administered by machines.
Deep learning can be used for supervised, unsupervised as well as reinforcement machine learning. it uses a variety of ways to process these.
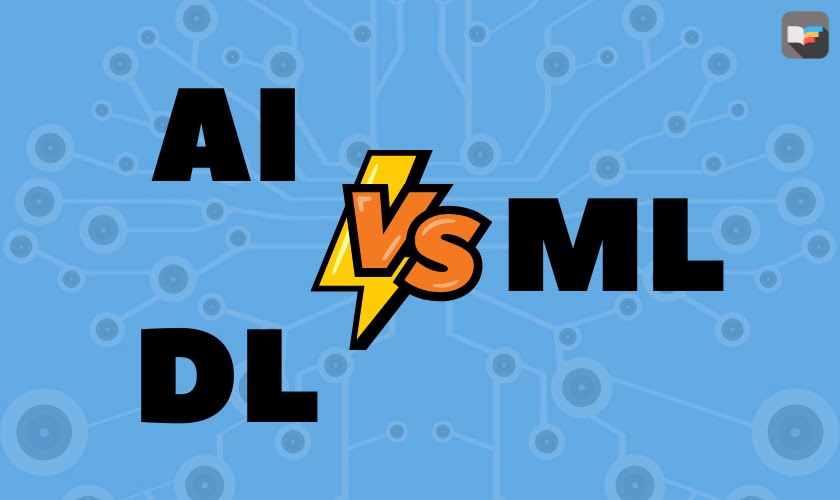
Difference between AI vs ML vs DL
Below is a table of differences between AI vs ML vs DL:
Artificial Intelligence | Machine Learning | Deep Learning |
---|---|---|
AI stands for Artificial Intelligence. | ML stands for Machine Learning. | DL stands for Deep Learning. |
Artificial Intelligence is basically the study/process which enables machines to mimic human behaviour through a particular algorithm. | Machine Learning is the study that uses statistical methods enabling machines to improve with experience. | Machine Learning is the study that uses statistical methods to enable machines to improve with experience. |
AI is the broader family consisting of ML and DL as its components. | ML is the subset of AI. | DL is the subset of ML. |
AI is the broader family consisting of ML and DL as its components. | ML is an AI algorithm which allows systems to learn from data. | DL is an ML algorithm that uses deep(more than one layer) neural networks to analyze data and provide output accordingly. |
AI is a computer algorithm which exhibits intelligence through decision-making. | The aim is to increase accuracy not caring much about the success ratio. | It attains the highest rank in terms of accuracy when it is trained with a large amount of data. |
Three broad categories/types Of AI are 1. Artificial Narrow Intelligence (ANI), 2. Artificial General Intelligence (AGI) and 3. Artificial Super Intelligence (ASI) | Three broad categories/types Of ML are: 1. Supervised Learning, 2. Unsupervised Learning and 3. Reinforcement Learning | DL can be considered as neural networks with a large number of parameter layers lying in one of the four fundamental network architectures: 1. Unsupervised Pre-trained Networks, 2. Convolutional Neural Networks, 3. Recurrent Neural Networks and 4. Recursive Neural Networks |
The efficiency Of AI is basically the efficiency provided by ML and DL respectively. | Less efficient than DL as it can’t work for longer dimensions or higher amounts of data. | More powerful than ML as it can easily work for larger sets of data. |
Examples of AI applications include Google’s AI-Powered Predictions, Ridesharing Apps Like Uber and Lyft, Commercial Flights Use an AI Autopilot, etc. | Examples of ML applications include Virtual Personal Assistants: Siri, Alexa, Google, etc., Email Spam and Malware Filtering. | Examples of DL applications include Sentiment based news aggregation, Image analysis and caption generation, etc. |
AI vs ML vs DL Examples
Artificial Intelligence (AI) refers to the development of computer systems that can perform tasks that would normally require human intelligence.
Examples of Artificial intelligence
There are numerous examples of AI applications across various industries. Here are some common examples:
- Speech recognition
- Personalized recommendations
- Predictive maintenance
- Medical diagnosis
- Autonomous vehicles
- Virtual Personal Assistants (VPA) like Siri or Alexa
- Autonomous vehicles
- Fraud detection
- Image recognition
- Natural language processing
- Game-playing AI
Examples of Machine Learning
Machine Learning (ML) is a subset of Artificial Intelligence (AI) that involves the use of algorithms and statistical models to allow a computer system to “learn” from data and improve its performance over time, without being explicitly programmed to do so.
- Image recognition
- Speech recognition
- Natural language processing (NLP)
- Recommendation systems
- Sentiment analysis
- Predictive maintenance
- Spam filters in the email
- Recommendation systems
- Predictive maintenance
- Credit risk assessment
- Customer segmentation
- Fraud detection
- Speech recognition
Examples of Deep Learning
Deep Learning is a type of Machine Learning that uses artificial neural networks with multiple layers to learn and make decisions.
- Image and video recognition
- Generative models
- Autonomous vehicles
- Image classification
- Speech recognition
- Natural language processing
- Recommender systems
- Fraud detection
- Game-playing AI
- Time series forecasting